Note
Go to the end to download the full example code.
GMM Plan 1D
Illustration of the GMM plan for the Mixture Wasserstein between two GMM in 1D, as well as the two maps T_mean and T_rand. T_mean is the barycentric projection of the GMM coupling, and T_rand takes a random gaussian image between two components, according to the coupling and the GMMs. See [69] for details. .. [69] Delon, J., & Desolneux, A. (2020). A Wasserstein-type distance in the space of Gaussian mixture models. SIAM Journal on Imaging Sciences, 13(2), 936-970.
# Author: Eloi Tanguy <eloi.tanguy@u-paris>
# Remi Flamary <remi.flamary@polytehnique.edu>
# Julie Delon <julie.delon@math.cnrs.fr>
#
# License: MIT License
# sphinx_gallery_thumbnail_number = 1
import numpy as np
from ot.plot import plot1D_mat, rescale_for_imshow_plot
from ot.gmm import gmm_ot_plan_density, gmm_pdf, gmm_ot_apply_map
import matplotlib.pyplot as plt
Generate GMMOT plan plot it
ks = 2
kt = 3
d = 1
eps = 0.1
m_s = np.array([[1], [2]])
m_t = np.array([[3], [4.2], [5]])
C_s = np.array([[[0.05]], [[0.06]]])
C_t = np.array([[[0.03]], [[0.07]], [[0.04]]])
w_s = np.array([0.4, 0.6])
w_t = np.array([0.4, 0.2, 0.4])
n = 500
a_x, b_x = 0, 3
x = np.linspace(a_x, b_x, n)
a_y, b_y = 2, 6
y = np.linspace(a_y, b_y, n)
plan_density = gmm_ot_plan_density(
x[:, None], y[:, None], m_s, m_t, C_s, C_t, w_s, w_t, plan=None, atol=2e-2
)
a = gmm_pdf(x[:, None], m_s, C_s, w_s)
b = gmm_pdf(y[:, None], m_t, C_t, w_t)
plt.figure(figsize=(8, 8))
plot1D_mat(
a,
b,
plan_density,
title="GMM OT plan",
plot_style="xy",
a_label="Source distribution",
b_label="Target distribution",
)
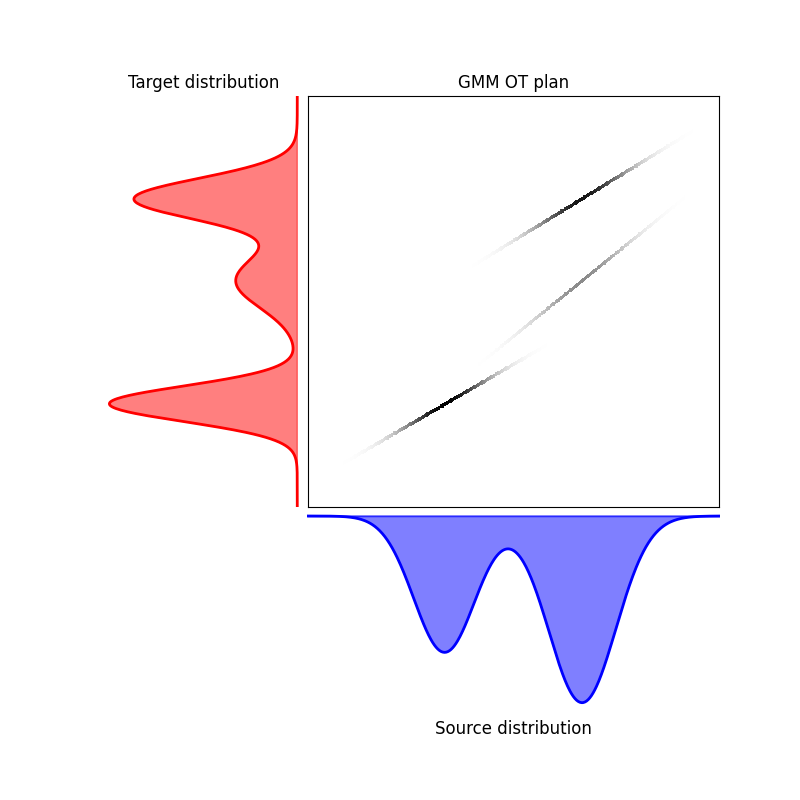
(<Axes: title={'center': 'Source distribution'}>, <Axes: title={'center': 'Target distribution'}>, <Axes: title={'center': 'GMM OT plan'}>)
Generate GMMOT maps and plot them over plan
plt.figure(figsize=(8, 8))
ax_s, ax_t, ax_M = plot1D_mat(
a,
b,
plan_density,
plot_style="xy",
title="GMM OT plan with T_mean and T_rand maps",
a_label="Source distribution",
b_label="Target distribution",
)
T_mean = gmm_ot_apply_map(x[:, None], m_s, m_t, C_s, C_t, w_s, w_t, method="bary")[:, 0]
x_rescaled, T_mean_rescaled = rescale_for_imshow_plot(x, T_mean, n, a_y=a_y, b_y=b_y)
ax_M.plot(
x_rescaled, T_mean_rescaled, label="T_mean", alpha=0.5, linewidth=5, color="aqua"
)
T_rand = gmm_ot_apply_map(
x[:, None], m_s, m_t, C_s, C_t, w_s, w_t, method="rand", seed=0
)[:, 0]
x_rescaled, T_rand_rescaled = rescale_for_imshow_plot(x, T_rand, n, a_y=a_y, b_y=b_y)
ax_M.scatter(
x_rescaled, T_rand_rescaled, label="T_rand", alpha=0.5, s=20, color="orange"
)
ax_M.legend(loc="upper left", fontsize=13)
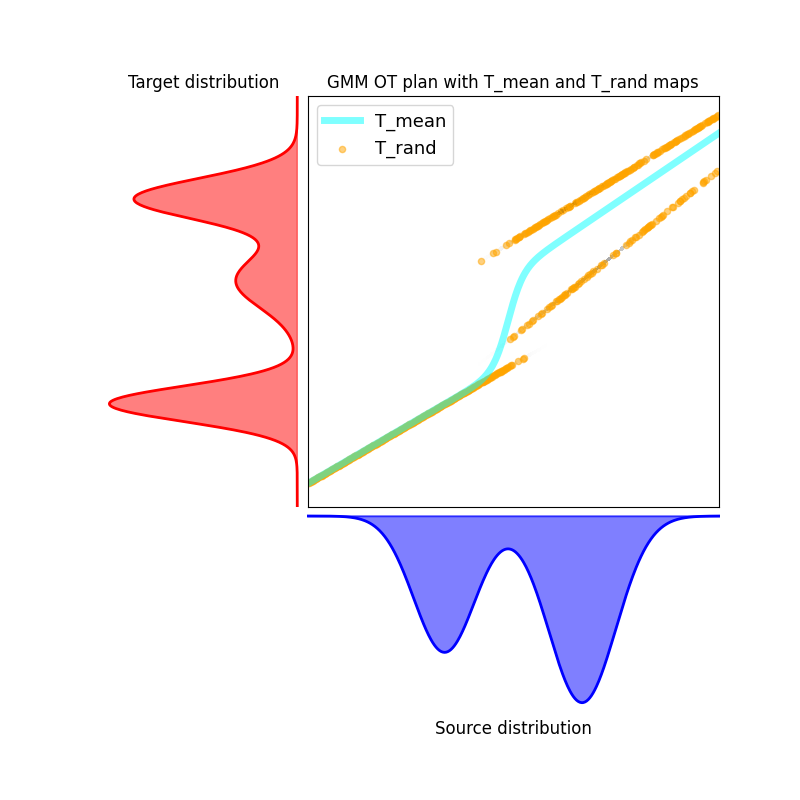
<matplotlib.legend.Legend object at 0x7ff964c49ab0>
Total running time of the script: (0 minutes 0.375 seconds)