Note
Go to the end to download the full example code.
Dual OT solvers for entropic and quadratic regularized OT with Pytorch
# Author: Remi Flamary <remi.flamary@polytechnique.edu>
#
# License: MIT License
# sphinx_gallery_thumbnail_number = 3
import numpy as np
import matplotlib.pyplot as pl
import torch
import ot
import ot.plot
Data generation
torch.manual_seed(1)
n_source_samples = 100
n_target_samples = 100
theta = 2 * np.pi / 20
noise_level = 0.1
Xs, ys = ot.datasets.make_data_classif(
'gaussrot', n_source_samples, nz=noise_level)
Xt, yt = ot.datasets.make_data_classif(
'gaussrot', n_target_samples, theta=theta, nz=noise_level)
# one of the target mode changes its variance (no linear mapping)
Xt[yt == 2] *= 3
Xt = Xt + 4
Plot data
pl.figure(1, (10, 5))
pl.clf()
pl.scatter(Xs[:, 0], Xs[:, 1], marker='+', label='Source samples')
pl.scatter(Xt[:, 0], Xt[:, 1], marker='o', label='Target samples')
pl.legend(loc=0)
pl.title('Source and target distributions')
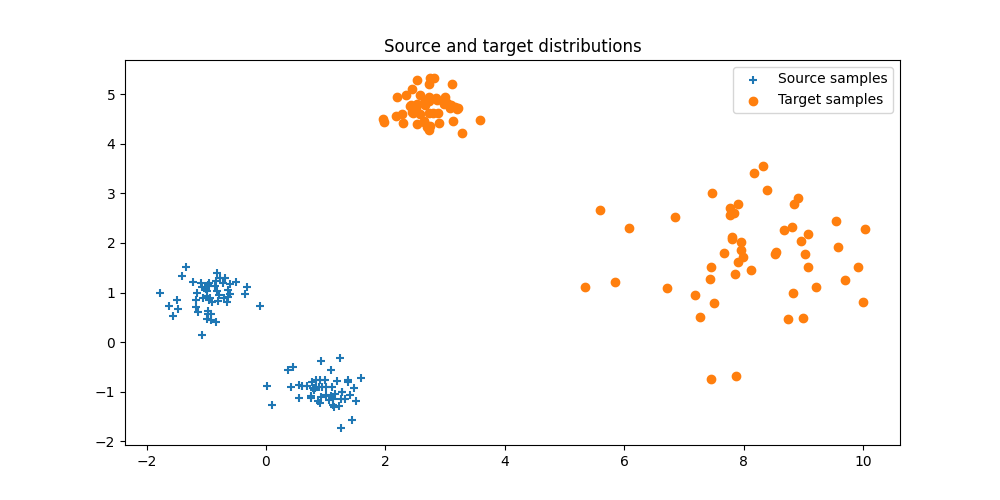
Text(0.5, 1.0, 'Source and target distributions')
Convert data to torch tensors
xs = torch.tensor(Xs)
xt = torch.tensor(Xt)
Estimating dual variables for entropic OT
u = torch.randn(n_source_samples, requires_grad=True)
v = torch.randn(n_source_samples, requires_grad=True)
reg = 0.5
optimizer = torch.optim.Adam([u, v], lr=1)
# number of iteration
n_iter = 200
losses = []
for i in range(n_iter):
# generate noise samples
# minus because we maximize te dual loss
loss = -ot.stochastic.loss_dual_entropic(u, v, xs, xt, reg=reg)
losses.append(float(loss.detach()))
if i % 10 == 0:
print("Iter: {:3d}, loss={}".format(i, losses[-1]))
loss.backward()
optimizer.step()
optimizer.zero_grad()
pl.figure(2)
pl.plot(losses)
pl.grid()
pl.title('Dual objective (negative)')
pl.xlabel("Iterations")
Ge = ot.stochastic.plan_dual_entropic(u, v, xs, xt, reg=reg)
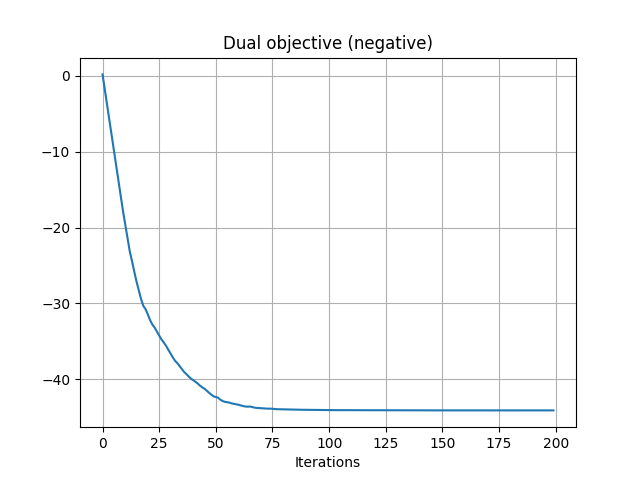
Iter: 0, loss=0.2020494900224819
Iter: 10, loss=-19.342886990309378
Iter: 20, loss=-31.04781266546102
Iter: 30, loss=-35.39220840550778
Iter: 40, loss=-38.45366508055631
Iter: 50, loss=-40.3977931689898
Iter: 60, loss=-41.11603809604154
Iter: 70, loss=-41.466702853376546
Iter: 80, loss=-41.58499551291181
Iter: 90, loss=-41.63288303069274
Iter: 100, loss=-41.65113903290441
Iter: 110, loss=-41.66139308084649
Iter: 120, loss=-41.66777613180863
Iter: 130, loss=-41.67121800015725
Iter: 140, loss=-41.67426045993204
Iter: 150, loss=-41.67679071343825
Iter: 160, loss=-41.67905002701037
Iter: 170, loss=-41.681095307214804
Iter: 180, loss=-41.68292759492431
Iter: 190, loss=-41.684535232401316
Plot the estimated entropic OT plan
pl.figure(3, (10, 5))
pl.clf()
ot.plot.plot2D_samples_mat(Xs, Xt, Ge.detach().numpy(), alpha=0.1)
pl.scatter(Xs[:, 0], Xs[:, 1], marker='+', label='Source samples', zorder=2)
pl.scatter(Xt[:, 0], Xt[:, 1], marker='o', label='Target samples', zorder=2)
pl.legend(loc=0)
pl.title('Source and target distributions')
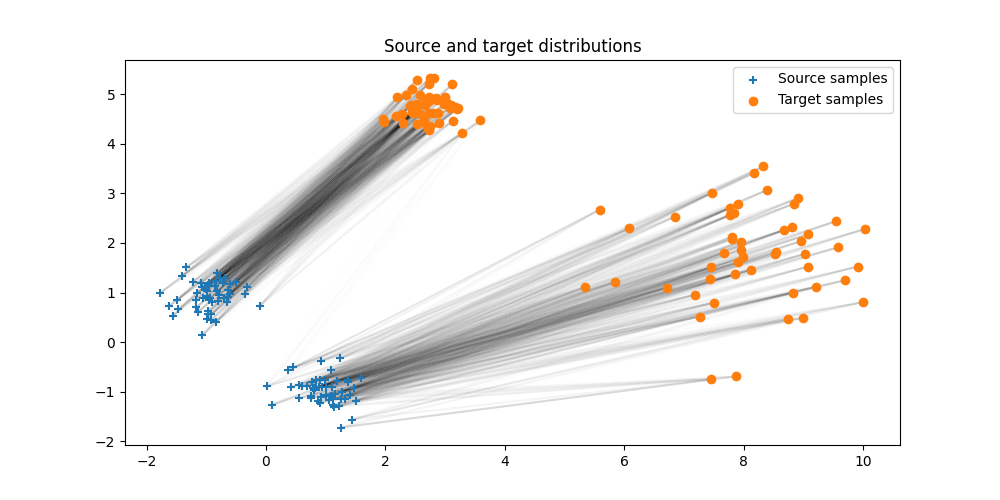
Text(0.5, 1.0, 'Source and target distributions')
Estimating dual variables for quadratic OT
u = torch.randn(n_source_samples, requires_grad=True)
v = torch.randn(n_source_samples, requires_grad=True)
reg = 0.01
optimizer = torch.optim.Adam([u, v], lr=1)
# number of iteration
n_iter = 200
losses = []
for i in range(n_iter):
# generate noise samples
# minus because we maximize te dual loss
loss = -ot.stochastic.loss_dual_quadratic(u, v, xs, xt, reg=reg)
losses.append(float(loss.detach()))
if i % 10 == 0:
print("Iter: {:3d}, loss={}".format(i, losses[-1]))
loss.backward()
optimizer.step()
optimizer.zero_grad()
pl.figure(4)
pl.plot(losses)
pl.grid()
pl.title('Dual objective (negative)')
pl.xlabel("Iterations")
Gq = ot.stochastic.plan_dual_quadratic(u, v, xs, xt, reg=reg)
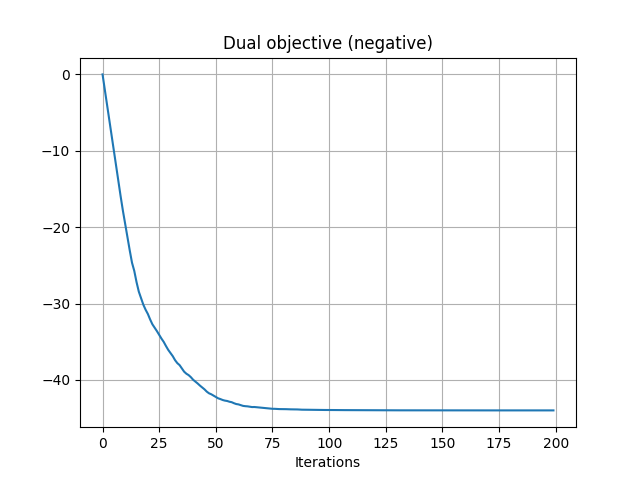
Iter: 0, loss=-0.0018442196020623663
Iter: 10, loss=-19.452586697782195
Iter: 20, loss=-30.798981324343657
Iter: 30, loss=-35.104119012236616
Iter: 40, loss=-38.417755073284575
Iter: 50, loss=-40.18296238061703
Iter: 60, loss=-40.96512264901174
Iter: 70, loss=-41.311650147302565
Iter: 80, loss=-41.41032726691648
Iter: 90, loss=-41.47884202567962
Iter: 100, loss=-41.50577883814947
Iter: 110, loss=-41.518992586502215
Iter: 120, loss=-41.52547606888991
Iter: 130, loss=-41.5292141960328
Iter: 140, loss=-41.53184270795394
Iter: 150, loss=-41.53407122063432
Iter: 160, loss=-41.5362397270994
Iter: 170, loss=-41.53841934532191
Iter: 180, loss=-41.54065142528501
Iter: 190, loss=-41.542916860389845
Plot the estimated quadratic OT plan
pl.figure(5, (10, 5))
pl.clf()
ot.plot.plot2D_samples_mat(Xs, Xt, Gq.detach().numpy(), alpha=0.1)
pl.scatter(Xs[:, 0], Xs[:, 1], marker='+', label='Source samples', zorder=2)
pl.scatter(Xt[:, 0], Xt[:, 1], marker='o', label='Target samples', zorder=2)
pl.legend(loc=0)
pl.title('OT plan with quadratic regularization')
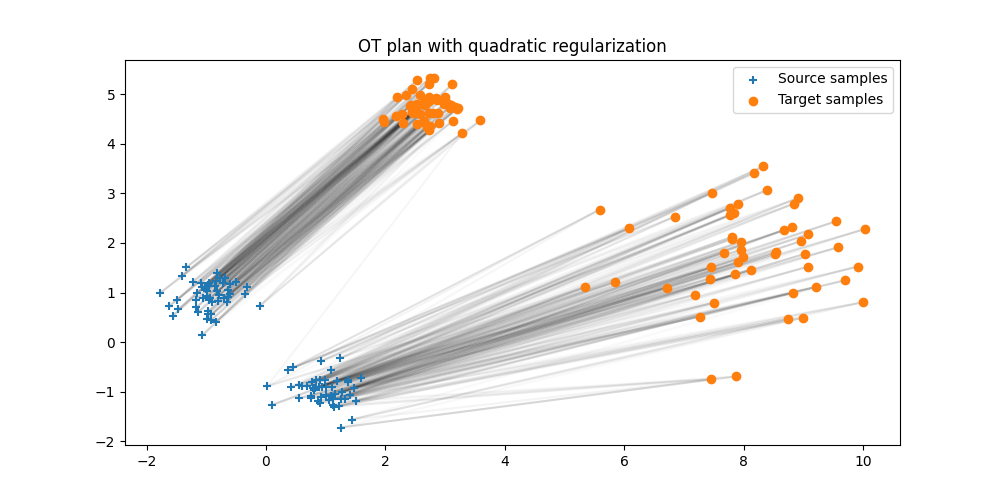
Text(0.5, 1.0, 'OT plan with quadratic regularization')
Total running time of the script: (0 minutes 11.931 seconds)