Note
Go to the end to download the full example code.
Smooth and Strongly Convex Nearest Brenier Potentials
This example is designed to show how to use SSNB [58] in POT. SSNB computes an l-strongly convex potential \(\varphi\) with an L-Lipschitz gradient such that \(\nabla \varphi \# \mu \approx \nu\). This regularity can be enforced only on the components of a partition of the ambient space, which is a relaxation compared to imposing global regularity.
In this example, we consider a source measure \(\mu_s\) which is the uniform measure on the unit square in \(\mathbb{R}^2\), and the target measure \(\mu_t\) which is the image of \(\mu_x\) by \(T(x_1, x_2) = (x_1 + 2\mathrm{sign}(x_2), 2 * x_2)\). The map \(T\) is non-smooth, and we wish to approximate it using a “Brenier-style” map \(\nabla \varphi\) which is regular on the partition \(\lbrace x_1 <=0, x_1>0\rbrace\), which is well adapted to this particular dataset.
We represent the gradients of the “bounding potentials” \(\varphi_l, \varphi_u\) (from [59], Theorem 3.14), which bound any SSNB potential which is optimal in the sense of [58], Definition 1:
where \(\mathcal{F}\) is the space functions that are on every set \(E_k\) l-strongly convex with an L-Lipschitz gradient, given \((E_k)_{k \in [K]}\) a partition of the ambient source space.
We perform the optimisation on a low amount of fitting samples and with few iterations, since solving the SSNB problem is quite computationally expensive.
THIS EXAMPLE REQUIRES CVXPY
# Author: Eloi Tanguy <eloi.tanguy@u-paris.fr>
# License: MIT License
# sphinx_gallery_thumbnail_number = 3
import matplotlib.pyplot as plt
import numpy as np
import ot
Generating the fitting data
n_fitting_samples = 30
rng = np.random.RandomState(seed=0)
Xs = rng.uniform(-1, 1, size=(n_fitting_samples, 2))
Xs_classes = (Xs[:, 0] < 0).astype(int)
Xt = np.stack([Xs[:, 0] + 2 * np.sign(Xs[:, 0]), 2 * Xs[:, 1]], axis=-1)
plt.scatter(
Xs[Xs_classes == 0, 0], Xs[Xs_classes == 0, 1], c="blue", label="source class 0"
)
plt.scatter(
Xs[Xs_classes == 1, 0],
Xs[Xs_classes == 1, 1],
c="dodgerblue",
label="source class 1",
)
plt.scatter(Xt[:, 0], Xt[:, 1], c="red", label="target")
plt.axis("equal")
plt.title("Splitting sphere dataset")
plt.legend(loc="upper right")
plt.show()
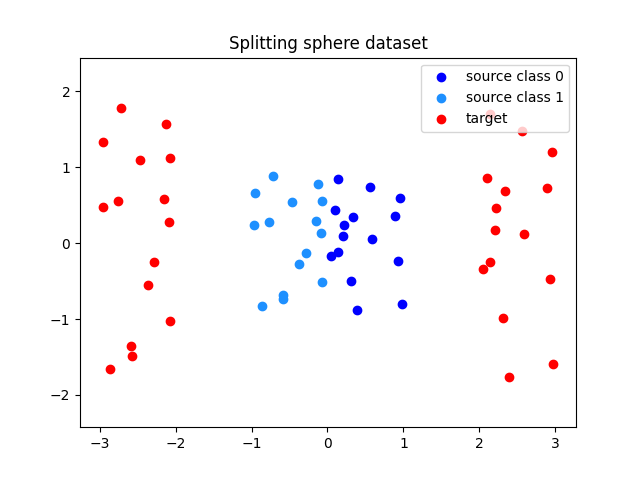
Fitting the Nearest Brenier Potential
L = 3 # need L > 2 to allow the 2*y term, default is 1.4
phi, G = ot.mapping.nearest_brenier_potential_fit(
Xs, Xt, Xs_classes, its=10, init_method="barycentric", gradient_lipschitz_constant=L
)
/home/circleci/.local/lib/python3.10/site-packages/cvxpy/reductions/solvers/solving_chain.py:356: FutureWarning:
You specified your problem should be solved by ECOS. Starting in
CXVPY 1.6.0, ECOS will no longer be installed by default with CVXPY.
Please either add ECOS as an explicit install dependency to your project
or switch to our new default solver, Clarabel, by either not specifying a
solver argument or specifying ``solver=cp.CLARABEL``. To suppress this
warning while continuing to use ECOS, you can filter this warning using
Python's ``warnings`` module until you are using 1.6.0.
warnings.warn(ECOS_DEP_DEPRECATION_MSG, FutureWarning)
Plotting the images of the source data
plt.clf()
plt.scatter(Xs[:, 0], Xs[:, 1], c="dodgerblue", label="source")
plt.scatter(Xt[:, 0], Xt[:, 1], c="red", label="target")
for i in range(n_fitting_samples):
plt.plot([Xs[i, 0], G[i, 0]], [Xs[i, 1], G[i, 1]], color="black", alpha=0.5)
plt.title("Images of in-data source samples by the fitted SSNB")
plt.legend(loc="upper right")
plt.axis("equal")
plt.show()
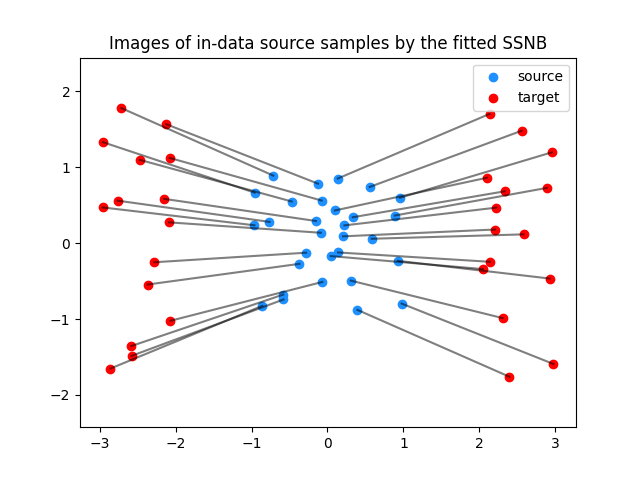
Computing the predictions (images by nabla phi) for random samples of the source distribution
n_predict_samples = 50
Ys = rng.uniform(-1, 1, size=(n_predict_samples, 2))
Ys_classes = (Ys[:, 0] < 0).astype(int)
phi_lu, G_lu = ot.mapping.nearest_brenier_potential_predict_bounds(
Xs, phi, G, Ys, Xs_classes, Ys_classes, gradient_lipschitz_constant=L
)
Plot predictions for the gradient of the lower-bounding potential
plt.clf()
plt.scatter(Xs[:, 0], Xs[:, 1], c="dodgerblue", label="source")
plt.scatter(Xt[:, 0], Xt[:, 1], c="red", label="target")
for i in range(n_predict_samples):
plt.plot(
[Ys[i, 0], G_lu[0, i, 0]], [Ys[i, 1], G_lu[0, i, 1]], color="black", alpha=0.5
)
plt.title("Images of new source samples by $\\nabla \\varphi_l$")
plt.legend(loc="upper right")
plt.axis("equal")
plt.show()
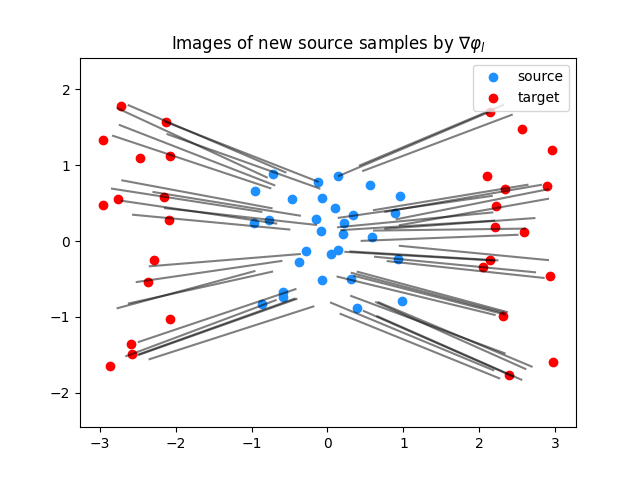
Plot predictions for the gradient of the upper-bounding potential
plt.clf()
plt.scatter(Xs[:, 0], Xs[:, 1], c="dodgerblue", label="source")
plt.scatter(Xt[:, 0], Xt[:, 1], c="red", label="target")
for i in range(n_predict_samples):
plt.plot(
[Ys[i, 0], G_lu[1, i, 0]], [Ys[i, 1], G_lu[1, i, 1]], color="black", alpha=0.5
)
plt.title("Images of new source samples by $\\nabla \\varphi_u$")
plt.legend(loc="upper right")
plt.axis("equal")
plt.show()
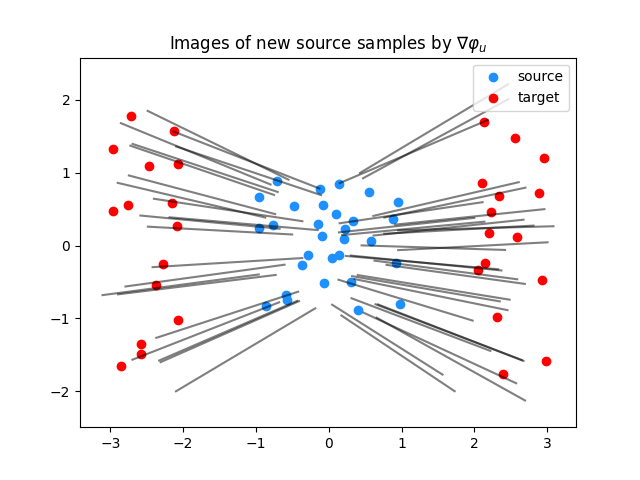
Total running time of the script: (0 minutes 47.016 seconds)