Note
Go to the end to download the full example code
Sliced Wasserstein barycenter and gradient flow with PyTorch
In this example we use the pytorch backend to optimize the sliced Wasserstein loss between two empirical distributions [31].
In the first example one we perform a gradient flow on the support of a distribution that minimize the sliced Wasserstein distance as proposed in [36].
In the second example we optimize with a gradient descent the sliced Wasserstein barycenter between two distributions as in [31].
[31] Bonneel, Nicolas, et al. “Sliced and radon wasserstein barycenters of measures.” Journal of Mathematical Imaging and Vision 51.1 (2015): 22-45
[36] Liutkus, A., Simsekli, U., Majewski, S., Durmus, A., & Stöter, F. R. (2019, May). Sliced-Wasserstein flows: Nonparametric generative modeling via optimal transport and diffusions. In International Conference on Machine Learning (pp. 4104-4113). PMLR.
# Author: Rémi Flamary <remi.flamary@polytechnique.edu>
#
# License: MIT License
# sphinx_gallery_thumbnail_number = 4
Loading the data
import numpy as np
import matplotlib.pylab as pl
import torch
import ot
import matplotlib.animation as animation
I1 = pl.imread('../../data/redcross.png').astype(np.float64)[::5, ::5, 2]
I2 = pl.imread('../../data/tooth.png').astype(np.float64)[::5, ::5, 2]
sz = I2.shape[0]
XX, YY = np.meshgrid(np.arange(sz), np.arange(sz))
x1 = np.stack((XX[I1 == 0], YY[I1 == 0]), 1) * 1.0
x2 = np.stack((XX[I2 == 0] + 60, -YY[I2 == 0] + 32), 1) * 1.0
x3 = np.stack((XX[I2 == 0], -YY[I2 == 0] + 32), 1) * 1.0
pl.figure(1, (8, 4))
pl.scatter(x1[:, 0], x1[:, 1], alpha=0.5)
pl.scatter(x2[:, 0], x2[:, 1], alpha=0.5)
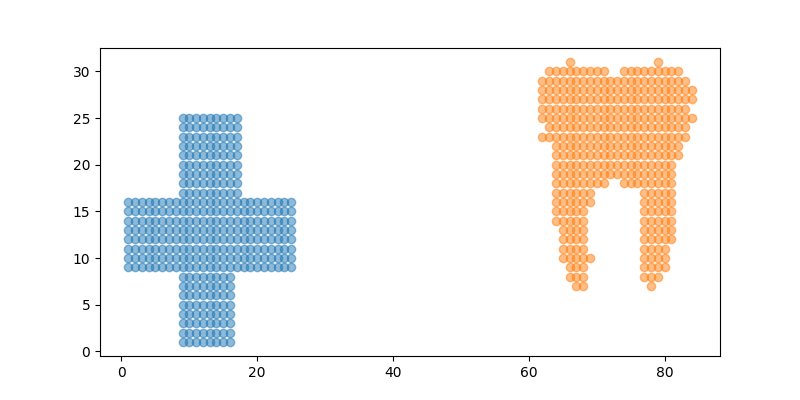
<matplotlib.collections.PathCollection object at 0x7f49df593b50>
Sliced Wasserstein gradient flow with Pytorch
device = "cuda" if torch.cuda.is_available() else "cpu"
# use pyTorch for our data
x1_torch = torch.tensor(x1).to(device=device).requires_grad_(True)
x2_torch = torch.tensor(x2).to(device=device)
lr = 1e3
nb_iter_max = 50
x_all = np.zeros((nb_iter_max, x1.shape[0], 2))
loss_iter = []
# generator for random permutations
gen = torch.Generator(device=device)
gen.manual_seed(42)
for i in range(nb_iter_max):
loss = ot.sliced_wasserstein_distance(x1_torch, x2_torch, n_projections=20, seed=gen)
loss_iter.append(loss.clone().detach().cpu().numpy())
loss.backward()
# performs a step of projected gradient descent
with torch.no_grad():
grad = x1_torch.grad
x1_torch -= grad * lr / (1 + i / 5e1) # step
x1_torch.grad.zero_()
x_all[i, :, :] = x1_torch.clone().detach().cpu().numpy()
xb = x1_torch.clone().detach().cpu().numpy()
pl.figure(2, (8, 4))
pl.scatter(x1[:, 0], x1[:, 1], alpha=0.5, label='$\mu^{(0)}$')
pl.scatter(x2[:, 0], x2[:, 1], alpha=0.5, label=r'$\nu$')
pl.scatter(xb[:, 0], xb[:, 1], alpha=0.5, label='$\mu^{(100)}$')
pl.title('Sliced Wasserstein gradient flow')
pl.legend()
ax = pl.axis()
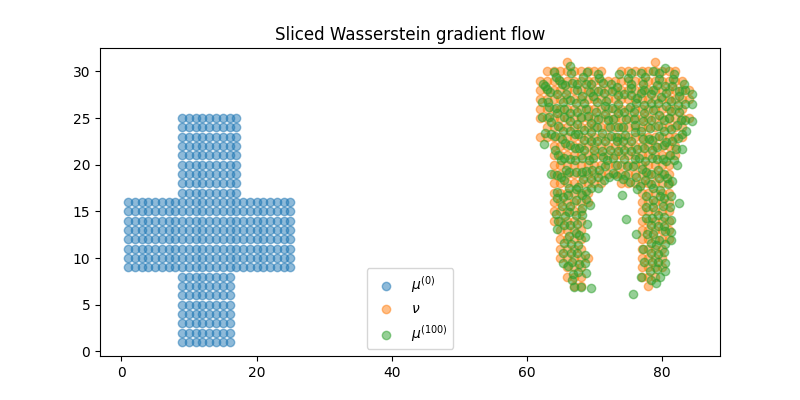
Animate trajectories of the gradient flow along iteration
pl.figure(3, (8, 4))
def _update_plot(i):
pl.clf()
pl.scatter(x1[:, 0], x1[:, 1], alpha=0.5, label='$\mu^{(0)}$')
pl.scatter(x2[:, 0], x2[:, 1], alpha=0.5, label=r'$\nu$')
pl.scatter(x_all[i, :, 0], x_all[i, :, 1], alpha=0.5, label='$\mu^{(100)}$')
pl.title('Sliced Wasserstein gradient flow Iter. {}'.format(i))
pl.axis(ax)
return 1
ani = animation.FuncAnimation(pl.gcf(), _update_plot, nb_iter_max, interval=100, repeat_delay=2000)
Compute the Sliced Wasserstein Barycenter
x1_torch = torch.tensor(x1).to(device=device)
x3_torch = torch.tensor(x3).to(device=device)
xbinit = np.random.randn(500, 2) * 10 + 16
xbary_torch = torch.tensor(xbinit).to(device=device).requires_grad_(True)
lr = 1e3
nb_iter_max = 50
x_all = np.zeros((nb_iter_max, xbary_torch.shape[0], 2))
loss_iter = []
# generator for random permutations
gen = torch.Generator(device=device)
gen.manual_seed(42)
alpha = 0.5
for i in range(nb_iter_max):
loss = alpha * ot.sliced_wasserstein_distance(xbary_torch, x3_torch, n_projections=50, seed=gen) \
+ (1 - alpha) * ot.sliced_wasserstein_distance(xbary_torch, x1_torch, n_projections=50, seed=gen)
loss_iter.append(loss.clone().detach().cpu().numpy())
loss.backward()
# performs a step of projected gradient descent
with torch.no_grad():
grad = xbary_torch.grad
xbary_torch -= grad * lr # / (1 + i / 5e1) # step
xbary_torch.grad.zero_()
x_all[i, :, :] = xbary_torch.clone().detach().cpu().numpy()
xb = xbary_torch.clone().detach().cpu().numpy()
pl.figure(4, (8, 4))
pl.scatter(x1[:, 0], x1[:, 1], alpha=0.5, label='$\mu$')
pl.scatter(x2[:, 0], x2[:, 1], alpha=0.5, label=r'$\nu$')
pl.scatter(xb[:, 0] + 30, xb[:, 1], alpha=0.5, label='Barycenter')
pl.title('Sliced Wasserstein barycenter')
pl.legend()
ax = pl.axis()
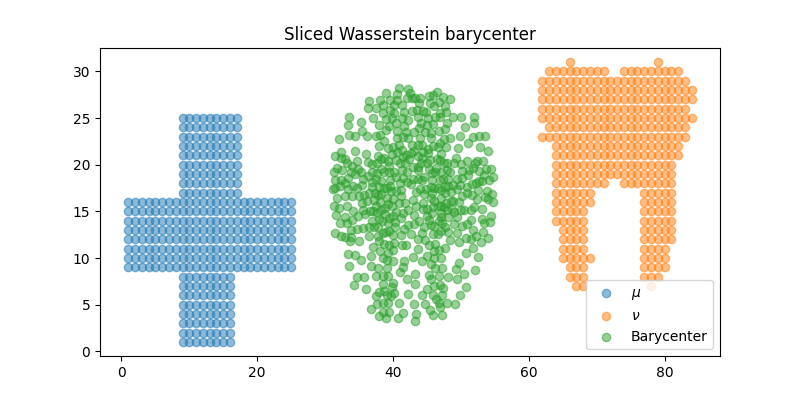
Animate trajectories of the barycenter along gradient descent
pl.figure(5, (8, 4))
def _update_plot(i):
pl.clf()
pl.scatter(x1[:, 0], x1[:, 1], alpha=0.5, label='$\mu^{(0)}$')
pl.scatter(x2[:, 0], x2[:, 1], alpha=0.5, label=r'$\nu$')
pl.scatter(x_all[i, :, 0] + 30, x_all[i, :, 1], alpha=0.5, label='$\mu^{(100)}$')
pl.title('Sliced Wasserstein barycenter Iter. {}'.format(i))
pl.axis(ax)
return 1
ani = animation.FuncAnimation(pl.gcf(), _update_plot, nb_iter_max, interval=100, repeat_delay=2000)
Total running time of the script: (0 minutes 46.473 seconds)